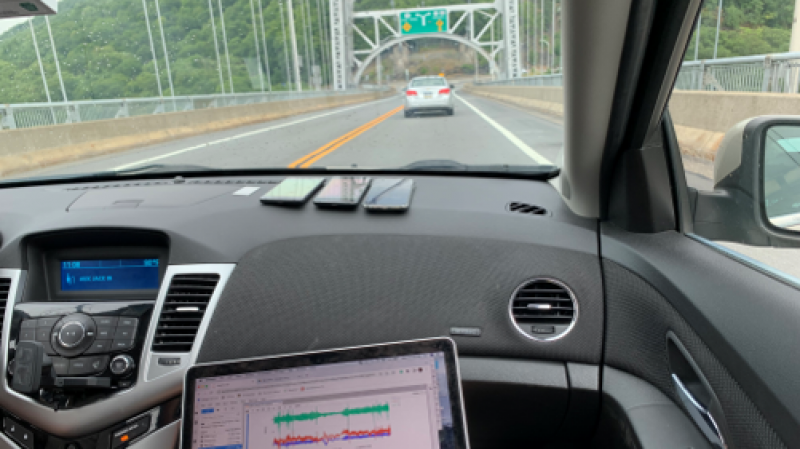
Bridges undergo significant vibrations and repeated stress variations during their operational life. Fatigue cracking and eventual fatigue failure of bridges are possible outcomes, highlighting the need for scalable life cycle analysis to estimate the remaining useful life of a structure accurately.
Fatigue life is typically estimated by the number of stress cycles a structure can withstand before failure. Engineers traditionally use strain gauges—sensors that measure changes in force or stress applied to an object—to collect strain measurements and assess the fatigue life of bridges.
However, large-scale deployment of wired strain gauges poses several limiting factors: it can be expensive, requires significant labor and material resources, and often requires additional spatial data. To overcome these constraints, engineers would benefit from a sensing strategy that supports information extraction from inexpensive data sources with higher spatial precision.
I-CPIE faculty member Shamim Pakzad, a professor in Lehigh University’s Department of Civil and Environmental Engineering, and his research team are using Pennsylvania Infrastructure Technology Alliance (PITA) funding to develop a novel approach to solve this challenge. In collaboration with the Center for Integrated Asset Management for Multimodal Transportation Infrastructure Systems (CIAMTIS), a U.S. DOT Region 3 University Transportation Center, Pakzad’s project focuses on employing deep learning (or deep neural networks) with physics-based foundations to exploit sensed data from structural health monitoring applications.
“Deep learning algorithms are designed to learn from data,” explains Pakzad. “As such, their performance improves with more data, which is ideal for the proposed crowdsensing approach.”
Unlike strain, acceleration can be measured relatively inexpensively through mobile sensing, an area of significant interest across many engineering fields. Mobile sensing eliminates the spatially restrictive feature of a fixed sensing approach, such as the use of strain gauges.
This project uses a mobile phone application to collect acceleration and GPS data from drivers crossing a bridge. The research team captures acceleration information from a series of mobile sensors (mobile phones of multiple drivers) and uploads the data to a cloud server. The acquired data is aligned, categorized, and processed to train the deep learning framework. The deep learning framework is then used to estimate dynamic strain for complex bridge structures.
“This project utilizes acceleration data collected by mobile device users traveling across a bridge, which helps to provide up-to-date information on the structure’s condition in an efficient manner,” says Pakzad. “This data is then fed to a deep learning-based framework to estimate dynamic properties of the structure and, ultimately, the strain levels that heavy traffic can cause.”
Smart mobile sensing presents an intriguing opportunity to help engineers assess the fatigue life of bridge structures in a manner that is more efficient and less expensive than traditional approaches. Pakzad indicates that future work is required to generalize the deep learning framework and validate the data collection, including enhancing the existing networks that will enable the estimate of strains in other directions.