Dr. Michael Kokkolaras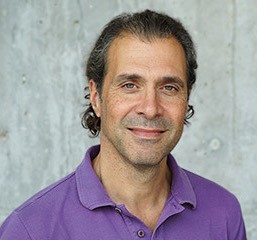
Professor and Associate Dean for Faculty Affairs
Department of Mechanical Engineering
McGill University
About Dr. Michael Kokkolaras
Dr. Michael Kokkolaras is Professor of Mechanical Engineering at McGill University, where he also serves as the Associate Dean for Faculty Affairs at the Faculty of Engineering. His research interests lie in the broad area of computational engineering design and include multidisciplinary, derivative-free, and Bayesian optimization; multi-fidelity model management, digital twins, and automated machine learning; requirements management; verification, validation, and uncertainty quantification. Dr. Kokkolaras is a member of a multi-university “Group for Research in Decision Analysis” (funded by the province of Quebec) and Visiting Professor at the Department of Industrial and Materials Science of Chalmers University of Technology in Sweden. He is an ASME Fellow, an Associate Fellow of the AIAA (serving on its Multidisciplinary Design Optimization Technical Committee), and elected member of the Advisory Board of the Design Society. Dr. Kokkolaras is currently serving as Publications Chair of the ASME Design Engineering Division (DED) and on the Advisory Board of the ASME Journal of Mechanical Design (JMD). He has previously served as: Associate Editor for the ASME JMD, Structural and Multidisciplinary Optimization (Springer), and Optimization and Engineering (Springer); Chair of the Design Automation Executive Committee of the ASME DED; Program and Conference Chair of the ASME Design Automation Conference; and Program Co-Chair of the International Conference on Engineering Design. Among other honors, he has received the ASME DED Design Automation Award, the University of Michigan College of Engineering Outstanding Research Scientist Award, and the ASME Journal of Mechanical Design Editors’ Choice Award.
Abstract
Computational design of engineering systems can entail several challenges. For example, requirements may change over time, especially for systems or components with relatively long lives; subsystem interactions need to be accounted for to ensure consistency; predictive models used to assess system behavior and performance typically exhibit fidelity-cost tradeoffs and are not always smooth.
In this talk, we will demonstrate that numerical optimization can be a useful tool in addressing such challenges. Specifically, we will present i) set-based optimization strategies for dealing with changing requirements, ii) an augmented Lagrangian method for coordinating distributed multidisciplinary optimization, and iii) a trust-region-based relative adequacy framework for managing the use of multi-fidelity models in design optimization problems. We rely on derivative-free optimization algorithms to solve complex non-smooth problems and use aerospace design applications to illustrate our work.
We will conclude by discussing ongoing and future research on Bayesian optimization, the combined algorithm selection and hyperparameter (CASH) optimization problem, and digital twins.