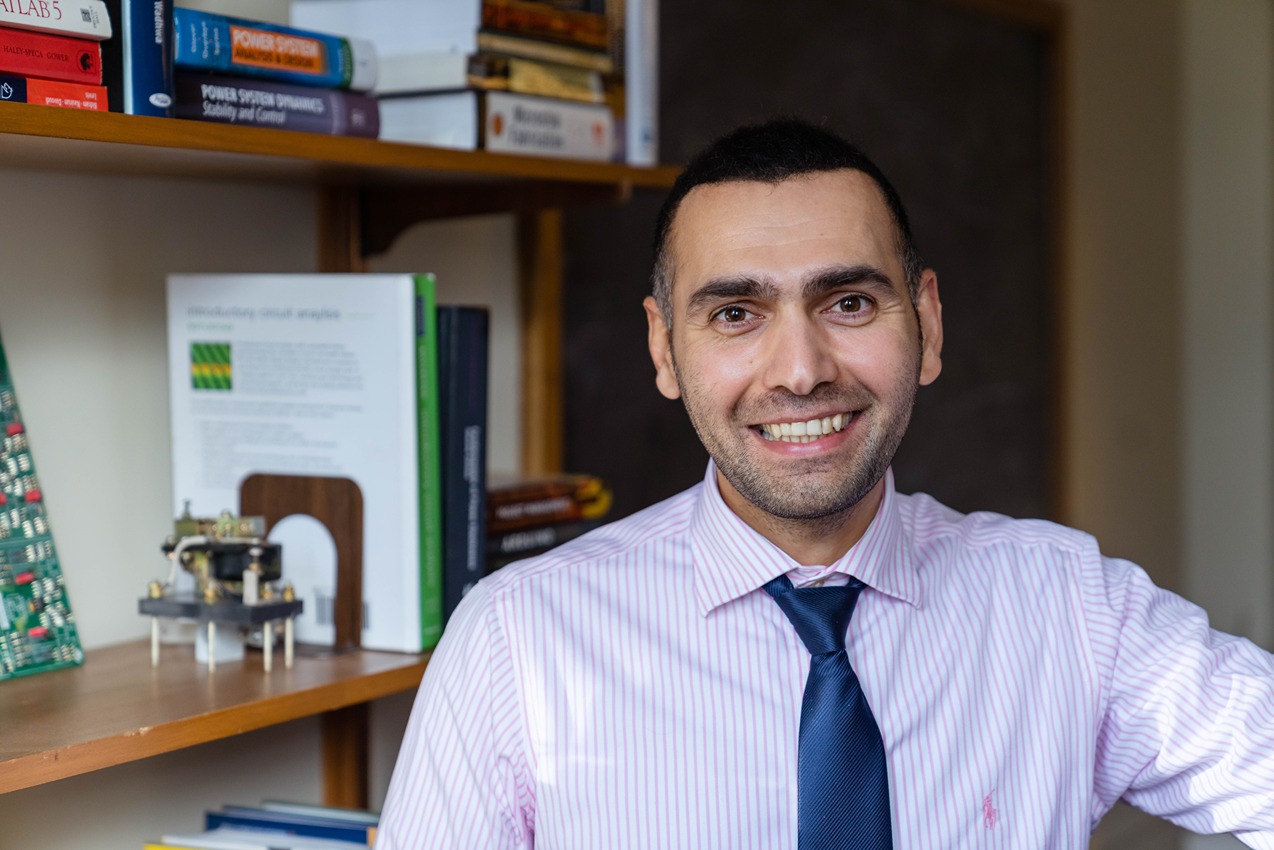
Lehigh University researcher Javad Khazaei has set a course to improve the way the Navy operates and maintains microgrids through the development of cutting-edge resilience assessment and control capabilities.
These small-scale grids, which can operate independently or collaboratively with other similar systems, power both the Navy’s ships and their onshore bases, says Khazaei, an assistant professor of electrical and computer engineering who leads the INTEGrated, Resilient and IntelligenT EnergY Systems (INTEGRITY) lab.
“We’re focused specifically on microgrids on DoD installations and floating microgrids on ships, which are composed of various electricity generation assets including generator sets, renewables, battery storage units, and supercapacitors, which together run everything on the DoD base or a ship. These systems are essential to our national security, but are decades old, and so the Navy wants to find a way to assess their operational resilience so they can determine if and when control design needs to be revisited or components should be replaced.”
This work on real-time microgrid operational resilience assessment is funded by a three-year, $546,929 award from the Office of Naval Research (ONR), one of two Department of Defense grants Khazaei has recently received. The second project, supported by a $970,850 award, focuses on optimizing microgrids in real-time using a deep-learning-based approach.
Monitoring resilience in real time
Khazaei says microgrid resilience assessments are typically done offline mainly because the resilience indexes that exist rely heavily on historical data. Such existing tools cannot provide real-time feedback on how the operational resilience changes over time, or if the resilience of a microgrid that has been operating for years is degrading. But he and his team are developing new operational resilience indexes using fundamental power system dynamic theories in time and frequency domain using voltage and frequency signals in real time, which will allow them to assess the operational resilience of every node within the system, and calculate a resilience index for each node.
A resilience index is a measure of how fast each element of the system could respond to a contingency (an unexpected failure of a single component due to a storm or an enemy attack), a fault (an instability in the system that occurs after bad weather, equipment failure, or a sudden change in demand), or a high-impact, low-frequency event (such as a tsunami).
The team will be applying the same concept to calculating resilience in unmanned Naval vehicles.
“Every microgrid has a fixed frequency and fixed voltage, and if they drop for any number of reasons, resilience is defined by how fast they return to their normal condition,” he says. “Our goal is to restore service to the ship as fast as possible, so it can continue the mission at maximum capacity or return safely back to its base after a major damage to the power and energy system of the ship (its main driver).”
Using real-time simulation models of Navy ships, the team will develop algorithms for each node of the microgrid and then conduct hardware-in-the-loop testing.
“We have a 10-kilowatt microgrid in our lab,” he says. “With this funding, we’ll add more capacity (12 kW) and new components to make a testbed that basically emulates a Navy ship. We’ll be able to then apply faults within this simulated environment, and see their impact on the actual hardware and assess the resilience in recovering from these faults.”
The team will also develop a dashboard that will monitor these resilience indexes in real time, and give microgrid operators recommendations on how to improve the systems when they go down.
“These recommendations could be upgrades on the components of a microgrid, modifications to the control design of generation assets and storage devices, or demand response designs. Then it will be up to the operator to determine which solution is suitable, for example, by identifying which load is not mission critical and can be turned off in order to restore the frequency and voltage back to the normal condition,” he says.
While grid resilience is directly tied to national security, Khazaei says another unique aspect to the project is its applicability across a range of systems—DC microgrids, AC microgrids, DC/AC hybrids, microgrids that are connected to the main grid, and those operating in islanded mode.
“This concept is generalized for any kind of energy system,” he says.
Tapping into the predictive power of machine learning
Khazaei’s second new ONR-funded project is a collaboration with Farrah Moazeni, an assistant professor of civil and environmental engineering who directs Lehigh’s interCONeccted Critical Infrastructure Systems Engineering (CONCISE) Laboratory.
The team will conduct research into deep-learning-based optimal control of naval microgrids. Moazeni will lead the efforts for designing a closed-loop model-based predictive control approach, and Khazaei will utilize these model-based designs to train a machine-learning-based controller that can run much faster than a model-based design.
The energy resources on a naval ship power a vast range of devices, the needs of which can change within microseconds.
“What we’re interested in learning is how we can use machine learning to optimally allocate resources in real time to ensure a successful mission,” says Khazaei.
Currently, he says, microgrid operators on ships use an open-loop structure. “They find out how many resources are available in real time and how much load they have, and then they run an optimization problem that minimizes the overall cost of running the system. This isn’t a resilient approach because it’s purely deterministic and doesn't consider uncertainties like bad weather, for example.” Closed-loop optimization techniques such as model predictive control can solve the challenge, but these approaches heavily rely on models and are computationally inefficient.
He and his team are using machine learning to formulate a closed-loop structure that enables predictive control.
“In the closed loop, your objective is minimizing cost, but you also consider the dynamic models of all these generators and assets in the formulation. So if I increase the speed of a ship at this moment, the power consumption of propulsion motors increases and the frequency of the microgrid in a ship might go down in the next couple of seconds. Using deep learning, our method will help predict the future behavior of each asset and enable better decision-making by telling you what the allocation of resources should be, and it will do that in milliseconds. For example, by increasing the reference power of a battery storage unit to respond to the frequency drop in real-time. So you’re minimizing cost, but you’re also improving the optimality and stability of the whole system. It’s called closed-loop optimization.”
Using advanced computers enhanced with multiple graphical processing units (GPUs), the team will develop the algorithms and then implement them on simulators of the ships before conducting hardware-in-the-loop testing. The next step will be demonstrating the concept on an actual ship.
The five-year project will eventually incorporate the resilience indexes Khazaei is developing in his other DOD initiative.
“We plan to implement those indexes into the deep-learning design, and eventually propose a machine-learning-based approach to resilience against failures.”
Research reported in this story is supported by the United States Navy/Office of Naval Research/Department of Defense, under award number N000142312402 and N000142312602.
Image credits: Christa Neu