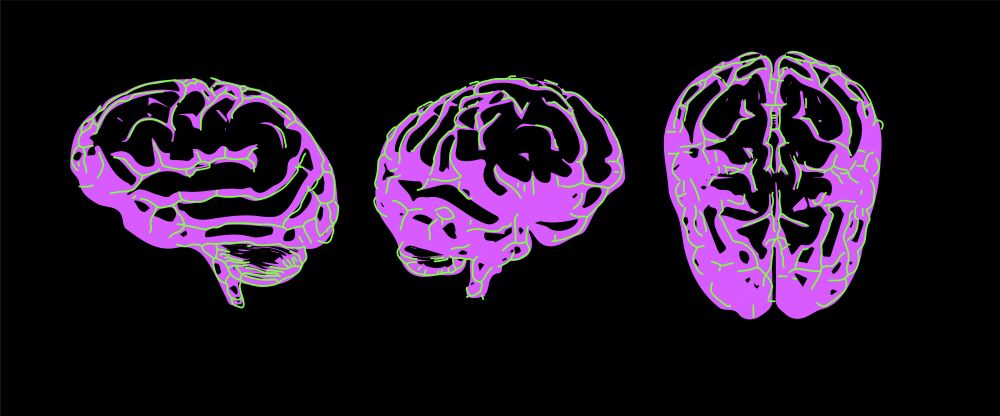
Brain networks are often represented by graph models that incorporate neuroimaging data from MRI or CT scans to represent functional or structural connections within the brain. These brain graphs can be used to understand how the organ changes over time.
Traditionally, however, these models treat the brain graphs as static, which can miss or ignore underlying changes that could signal the onset of disease or neurological disorders.
A collaborative team of researchers led by Lifang He, an assistant professor of computer science and engineering at Lehigh University, Liang Zhan, an associate professor of electrical and computer engineering at the University of Pittsburgh, and Carl J. Yang, an assistant professor of computer science at Emory University, recently received a $1 million grant from the National Science Foundation, with $300,000 going to Lehigh, to develop new methods for modeling the dynamics of brain graphs using artificial intelligence that will generate more accurate, interpretable, and fair predictions when it comes to disease.
“A static graph represents a single point in time,” says He. “In contrast, a dynamic graph represents multiple images taken at multiple points in time. We’ll be using a new AI method called neural ODE, or ordinary differential equation, to analyze these dynamic brain graphs. The ODE is adept at capturing dynamics in continuous time that could unveil the progression of disease.”
Neural ODE combines deep learning with concepts from ordinary differential equations, and allows a neural network to model both continuous change and sudden discontinuous change that can lead to more accurate predictions.
She says this AI tool will also facilitate the discovery of biomarkers—genetic traits, changes, or alterations associated with the brain—that can help detect disease or disorders.
“There are researchers focused only on disease prediction or biomarker discovery, but we’ll be focusing on both simultaneously,” she says.
The team will first extract brain graph data from neuroimaging scans and then develop a unified framework of Brain Graph Ordinary Differential Equations (BrainGDE) interweaving graph neural networks and ODEs to analyze the data, conduct the predictions, and identify which regions or biomarkers are associated with disease.
“This will allow us to track how disease develops in individual patients,” says He. “So it’s allowing for a more personalized approach to care.”
The project could help reshape deep learning techniques for temporal data mining in bioinformatics and healthcare technologies.
The approach, says He, “could revolutionize our approach to both diagnosis and treatment.”
About Lifang He
Lifang He is an assistant professor in the Department of Computer Science and Engineering in Lehigh University’s P.C. Rossin College of Engineering and Applied Science. Before her current role, she was a joint postdoctoral associate in the Department of Biostatistics, Epidemiology, and Informatics at the Perelman School of Medicine, University of Pennsylvania, and at the Weill Cornell Medical College of Cornell University. Dr. He's research group focuses on developing advanced computational methods for biomedical research, such as understanding disease mechanisms, diagnosis, prognosis, disease biomarkers, and disease pathways. Her research interests broadly include machine learning, computational medical imaging, AI for health, tensor computing, and multimodal analysis. Her research has been supported by several esteemed organizations, including NSF, NIH, and ONR. Dr. He currently serves as an associate editor for the Artificial Intelligence in Radiology section of the Frontiers journal and has been the Chair of the IEEE Computer Society Chapter at the Lehigh Valley Section since January 2023.