Student: Ben Kramer
Project: Implementing Machine Learning Based Reduced Order Modeling for Simulating Large Schools of Fish
Poster: Vertical (PDF) | Horizontal (PDF)
Institution: Lehigh University
Major: Mechanical Engineering and Mechanics
Advisor: Keith Moored
Abstract
With the exception of highly idealized models, computational methods are required to compute the fluid flow around and resulting forces acting on a body. However, traditional methods scale poorly to large scale simulations with computational costs increasing as the square of the number of elements being considered. To decrease time and computational costs, reduced order models (ROMs) are developed to replicate their full order model (FOM) counterparts. The Naval Research Lab (NRL) developed one such model that confers a 2000 times decrease in computing time while maintaining stability with a 0.1% error as compared to the FOM.
One application where a ROM is needed is in simulating the hydrodynamics of large fish schools. While models with a smaller number of swimmers can be constructed and evaluated, simulations with hundreds of swimmers cannot due to the computational limitations of current technology. The amount of computing power required to generate this simulation far exceeds the capabilities of most computers or takes excessive computing time on the world’s fastest supercomputers. Our work focuses on implementing the NRL-developed ROM into a boundary element solver capable of simulating the hydrodynamics of fish schools. This will lead to improved scaling capabilities of the solver and ultimately allow for large schools of hundreds of fish to be simulated.
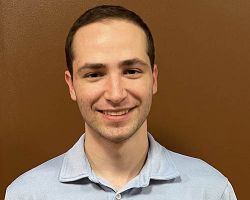
About Ben Kramer
Benjamin Kramer is a third-year undergraduate student at Lehigh University majoring in mechanical engineering and minoring in aerospace engineering. He is currently studying fluid mechanics with Professor Keith Moored and is focusing on simulating the fluid flows around bodies. Ben’s interests primarily include finite element analysis, computational fluid dynamics, machine learning, and control theory. He enjoys learning new concepts and implementing them into an existing method of solutions.