Resolve Magazine Fall 2023 >> Making Sense of Machine Learning
There’s recently been a surge of media coverage around machine learning and artificial intelligence (AI). And you would be forgiven for thinking they’re essentially the same thing, as the terms are often used interchangeably.
“They overlap, but for those of us who work in this space, they are distinct,” says professor Brian Davison, chair of the Department of Computer Science and Engineering. “AI is an umbrella term that encompasses any computing technique that seems intelligent, and one technique under that umbrella is machine learning.”
More specifically, artificial intelligence is the development of systems that are capable of mimicking human intelligence to perform tasks such as image, speech, and facial recognition; decision-making; and language translation. These systems are capable of iterating to improve their performance. Programmers and developers build them with tools that include machine learning, deep learning, neural networks, computer vision, and natural language processing—all of which allow the systems to simulate the reasoning humans use to learn, problem-solve, and make decisions.
As a subfield of AI, machine learning employs algorithms to enable computers to learn from data to identify patterns and then do something with that recognition, such as make a prediction, a decision, or a recommendation. Examples of artificial intelligence that uses machine learning are vast and include virtual assistants, navigational systems, recommendation systems (think Netflix), and chatbots like ChatGPT.
To some, however, words like intelligent and learning can be fraught.
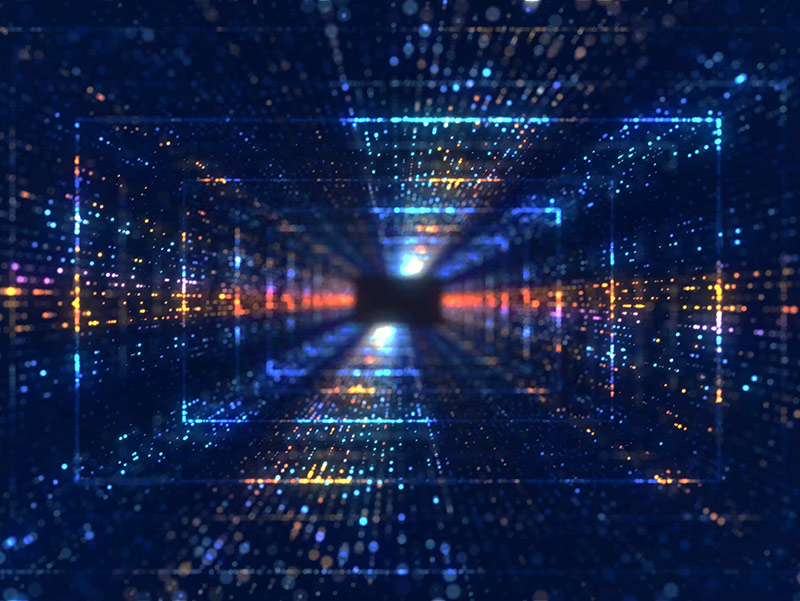
Diving deeper into artificial intelligence
Lehigh researchers engage in machine learning in myriad ways. Check out the expanded digital content availble via Resolve online, including:
➱ Designing Fair Algorithms: Designing to make predictions that aren’t influenced by data bias
➱ Exposing Fakes: Are you sure what you are seeing is real?
➱ Decoding disease: Using AI to identify the features associated with healthy and diseased tissue
➱ Protecting Diversity: Using machine learning to preserve and revive native languages
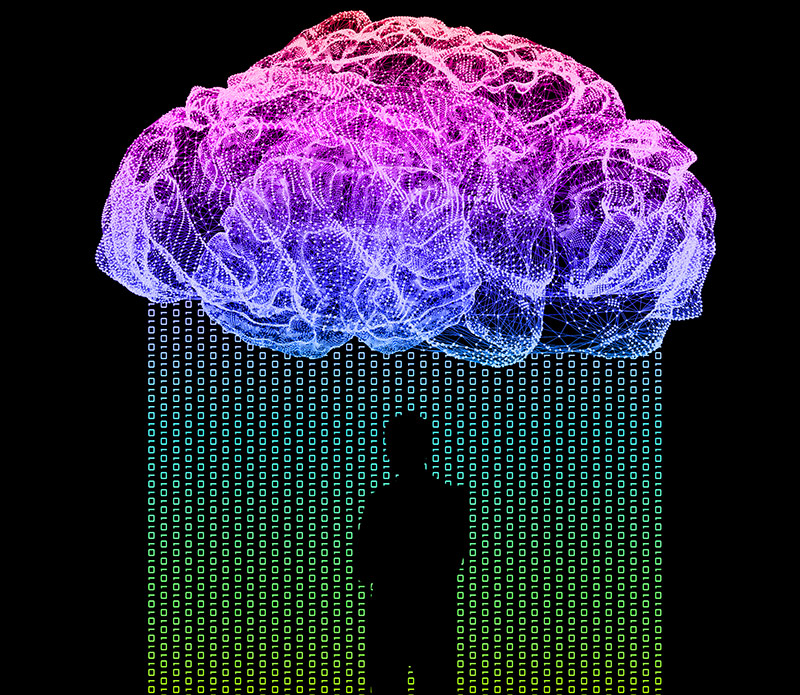
Clouded judgment: ethics, bias, and fairness in the age of AI
Why did so many of the experts who signed the "Statement of AI Risk" call their life’s work an "existential threat"? In part, it may be because they’ve released something into the world without fully understanding how it works.
"When you look at large language models (LLMs), like what powers ChatGPT, there are hundreds of billions of parameters," says Eric Baumer, an associate professor of computer science and engineering (CSE). "Once the model is trained, it’s so complex that it becomes difficult to interrogate and understand why any one of those billions of parameters influences the output."
So while the creators of these systems are knowledgeable about algorithms and the training process, he says, they don’t have a complete understanding. "And I think that’s what leads to these claims about them being an existential threat."