Resolve Magazine Fall 2023 >> Making Sense of Machine Learning >> Stories
Research teams from across the Rossin College are tackling challenges involving the fundamentals of machine learning and how the technology is being used in fields such as manufacturing, medicine, finance, and renewable energy. Their stories are just a sample of the innovative work on artificial intelligence taking place at Lehigh.
Read the article Making Sense of Machine Learning, Resolve Magazine, Fall 2023.
EXPANDED DIGITAL CONTENT:
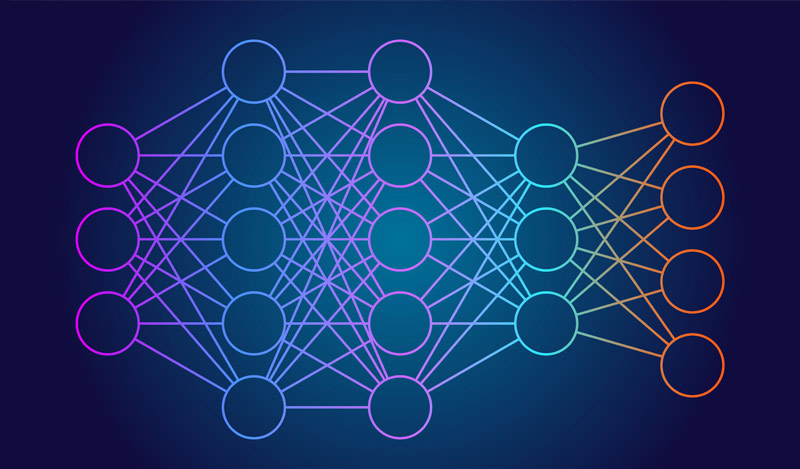
Designing fair algorithms
“There isn’t going to be a perfect algorithm because there isn’t going to be a perfect sense of what’s fair and what’s not,” says Lehigh industrial and systems engineering professor Frank Curtis. Curtis' research focuses on the design of algorithms—how to make them faster, more accurate, and more transferable across tasks. He’s also studying how to make them more fair. | READ MORE >>
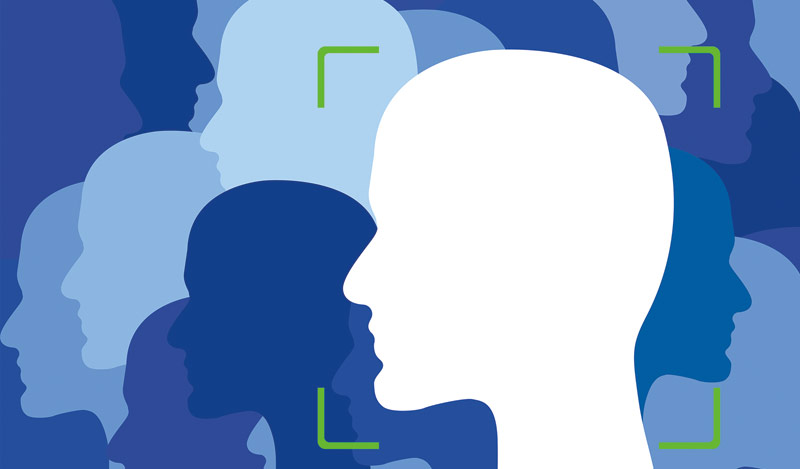
Computing fairer trade-offs
As machine learning is increasingly deployed across a range of sectors, mitigating bias and improving fairness in these systems has become a critical concern. But achieving both high levels of accuracy and fairness is an elusive goal, as algorithms are typically designed to maximize one or the other. | READ MORE >>
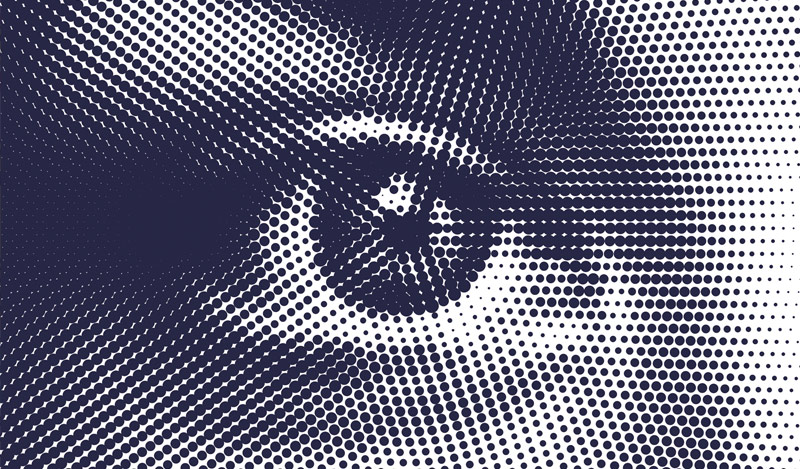
Exposing fakes
As you scroll through your social media feeds, are you sure what you’re seeing is real? “The human visual system is very advanced, and we can easily recognize real-world objects,” says Aparna Bharati, Lehigh assistant professor of computer science and engineering. “But online, we can very easily be fooled by high-quality fakes, and sometimes, even by the low-quality ones.” | READ MORE >>
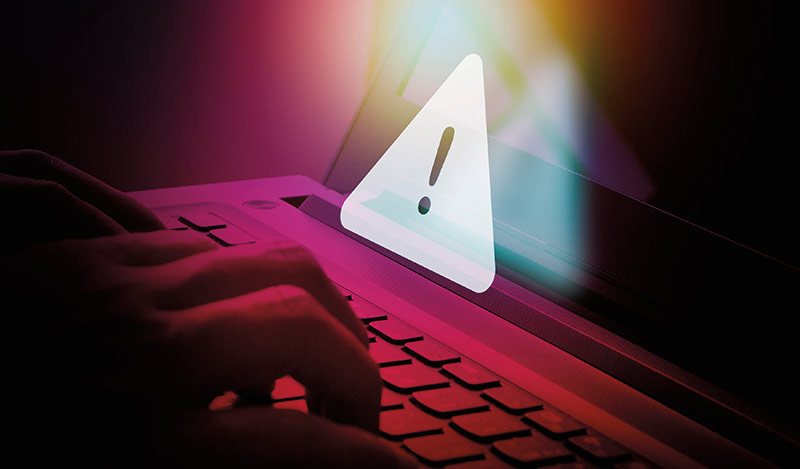
Evaluating trustworthiness
Large language models like ChatGPT are capable of recognizing and generating content in a very humanlike way. Which means they are ripe for bad behavior. Perhaps unsurprisingly, given how new the technology is, there hasn’t been much research into the ethical and moral compliance of LLMs, or in other words, their trustworthiness. | READ MORE >>
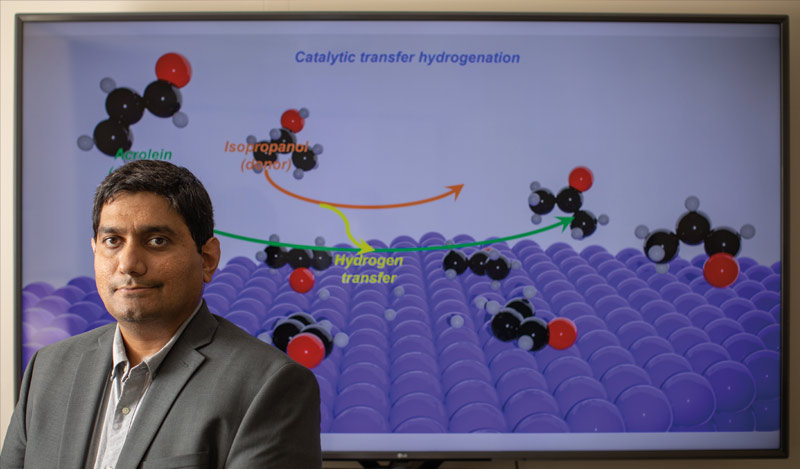
Building domain-informed models
Collaboration between those conducting foundational research into machine learning and those who apply machine learning methods is laying the groundwork at Lehigh for creating more precise tools to solve a range of compelling science and engineering problems. | READ MORE >>
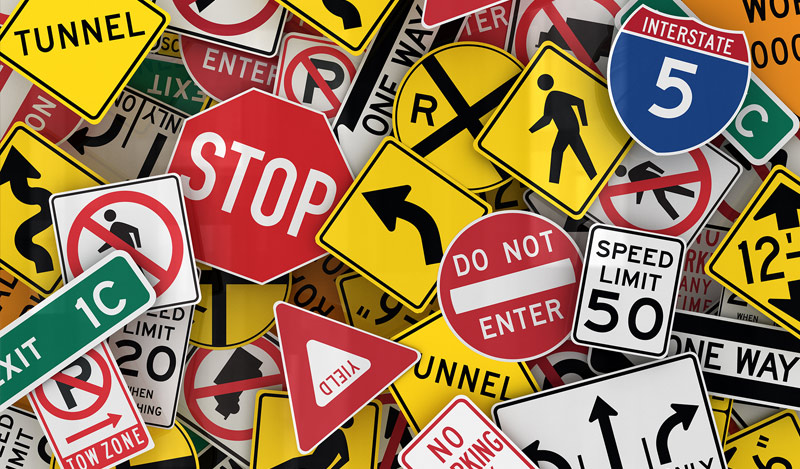
Solving ambiguity in robot perception
“For robots to achieve true autonomy in the future, they must be able to assess risks before making decisions,” says Nader Motee, a professor of mechanical engineering and mechanics. But a world with autonomous robots could be a world in which we humans get a lot of meaningful assistance from machines. | READ MORE >>
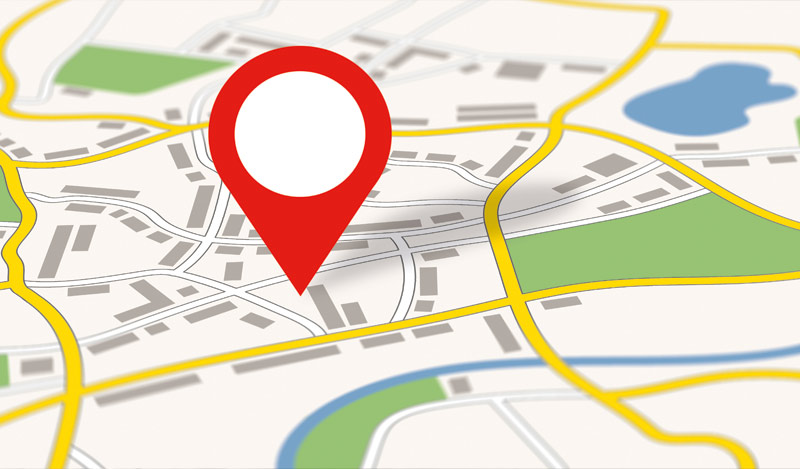
Tweaking the tools
A lot of us depend on GPS to get around. But what if there’s a hurricane or a wildfire and we have to immediately evacuate? Could we safely rely on those navigation systems? Professor Parv Venkitasubramaniam, electrical and computer engineering, and members of Lehigh’s Explainable Graph Learning research group are exploring this problem. | READ MORE >>
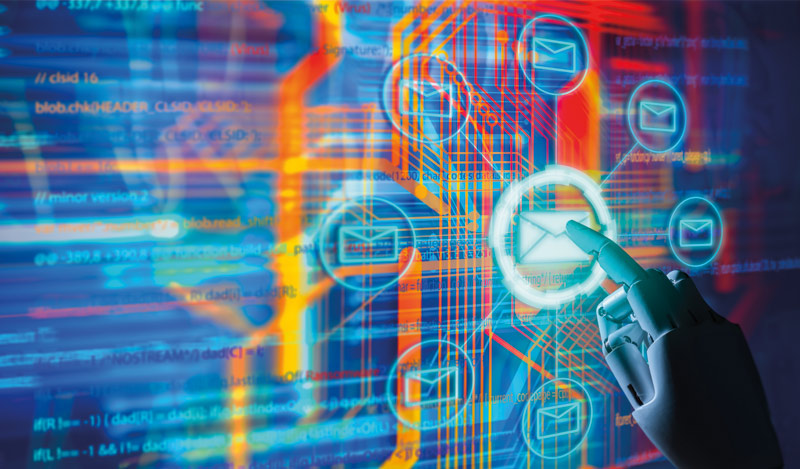
Subjecting models to humans
Before a machine learning model is released, it undergoes performance metrics. For many such models, those metrics include an F-score, which looks for a combined balance precision and recall in its results. “The problem,” says Lehigh associate professor Eric Baumer, “is that all false positives and all false negatives are treated equally and contribute to precision and recall in the same way.” | READ MORE >>
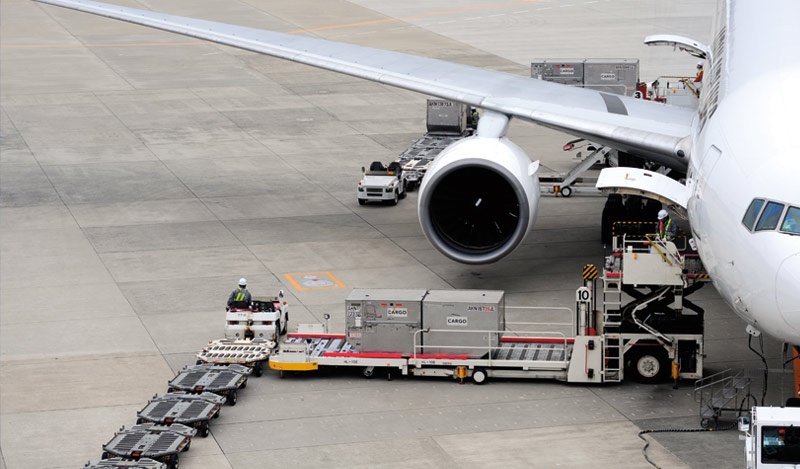
Guiding manufacturing principles
Sheet extrusion is a technique for making flat plastic sheets that are then cooled between rollers that exert high closing forces. Lehigh associate professor Ganesh Balasubramanian and his team are working in tandem with a Pennsylvania company to develop a machine-learning-informed strategy that can be integrated into the company’s sheet-extrusion platform. | READ MORE >>
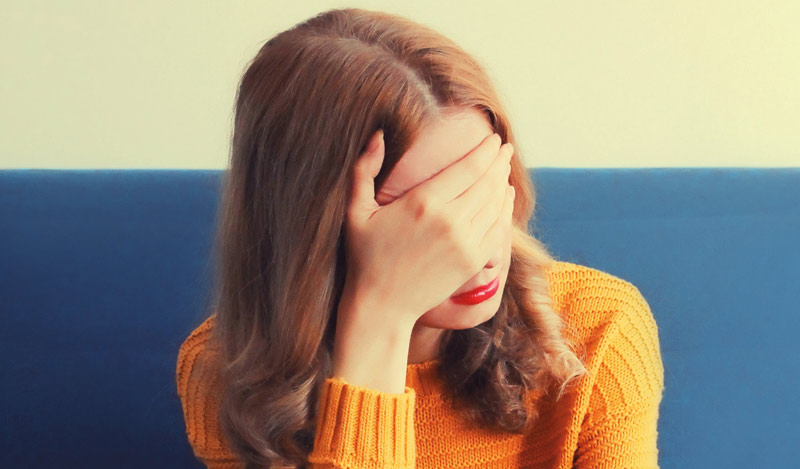
Decoding disease
Chronic fatigue syndrome, says Lehigh professor Xuanhong Cheng, is a difficult condition for doctors to diagnose, she says, because it’s unclear whether the symptoms are caused by a psychological or physiological issue. Cheng is part of a team using machine learning to measure the differences between cells from healthy and diseased muscle tissue. | READ MORE >>
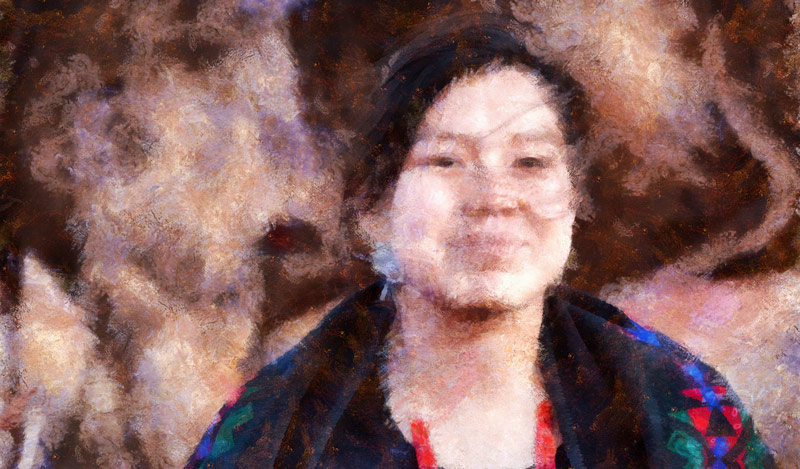
Protecting diversity
“Saving and revitalizing endangered languages is important for maintaining cultural diversity,” says associate professor Maryam Rahnemoonfar. She serves as a the faculty mentor for a Lehigh project using machine learning to revive the languages of Native Americans. A major hurdle is the lack of resources featuring available languages to feed the deep learning algorithms. | READ MORE >>
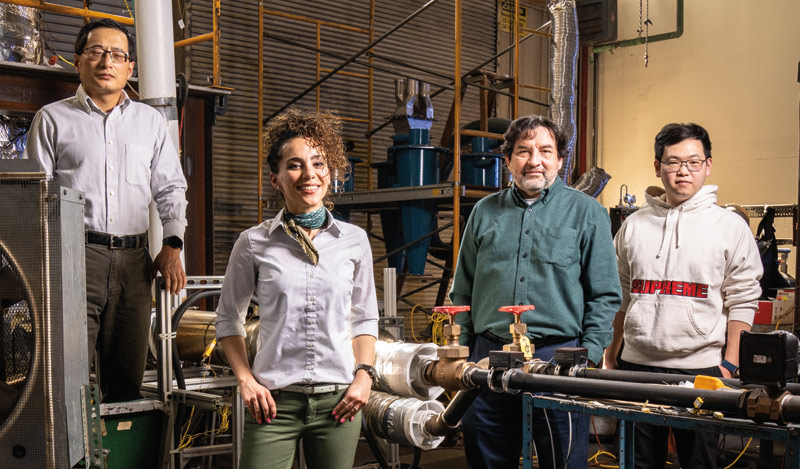
Making garbage greener
Shifting away from an economy based on fossil fuels will require, in part, a more efficient way of producing hydrogen, a clean fuel that yields an innocuous by-product: water. Lehigh Energy Research Director Carlos Romero is part of a team developing a machine learning approach to provide real-time solutions to get a “step closer to the hydrogen economy." | READ MORE >>
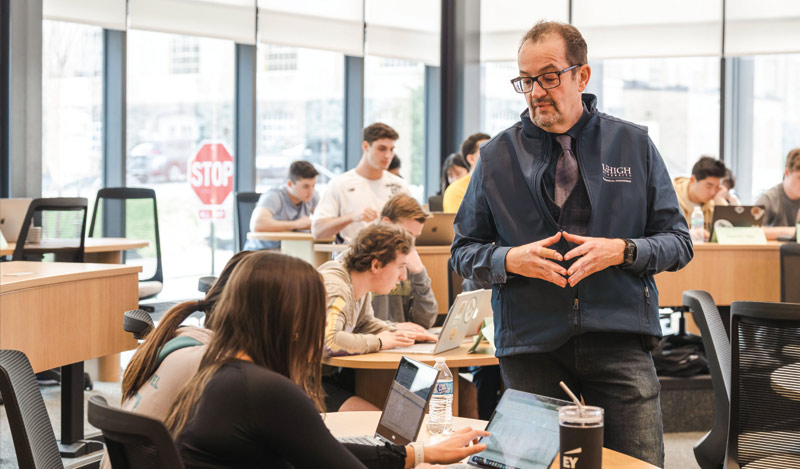
Preparing future professionals
Corporate-sponsored projects in machine learning are giving students in Lehigh’s MS in Financial Engineering (MFE) program an edge in the job market. Working with industry partners, students can build experience with technologies giving them an opportunity to differentiate themselves after graduation. | READ MORE >>