Stochastic Models and Optimization
Uncertainty is intrinsic in many science and engineering application domains, such as energy systems, supply chains, healthcare systems, manufacturing, disaster management, finance, and marketing. Therefore, the ability to address uncertainty accurately and robustly is essential for decisions that have been obtained through mathematical modeling and optimization to be reliable for real-world use. On a related note, various algorithms that have been designed for solving problems that can be represented by such models have also proved to be effective for solving certain (large-scale) deterministic problems for which classical algorithms have been found to falter.
Stochastic models that address real-world uncertainty are instrumental throughout industrial and systems engineering. Examples include stochastic programs, (distributionally) robust optimization models, scenario/decision trees, and discrete-event simulation models. Stochastic algorithmic methods, on the other hand, provide powerful computational tools for solving both stochastic and (large-scale) deterministic optimization and other types of problems. Examples include stochastic gradient methods to train machine learning models and probabilistic methods to approximately solve hard combinatorial optimization problems.
At Lehigh ISE, we advance the theoretical and computational frontiers of stochastic operations research by creating the next generation of data-driven stochastic models and methodologies relevant to optimization and decision-making under uncertainty. Our contributions span areas such as applied stochastic processes, stochastic programming, distribution-free optimization, uncertainty quantification, and stochastic and randomized optimization algorithms. In the past decade, our faculty have introduced new computationally efficient and data-driven stochastic optimization and related methodologies for solving numerous real-life problems in areas such as healthcare scheduling, facility location, transportation, time-series analysis, image classification, and speech recognition.
Lehigh's research groups / institutes / programs: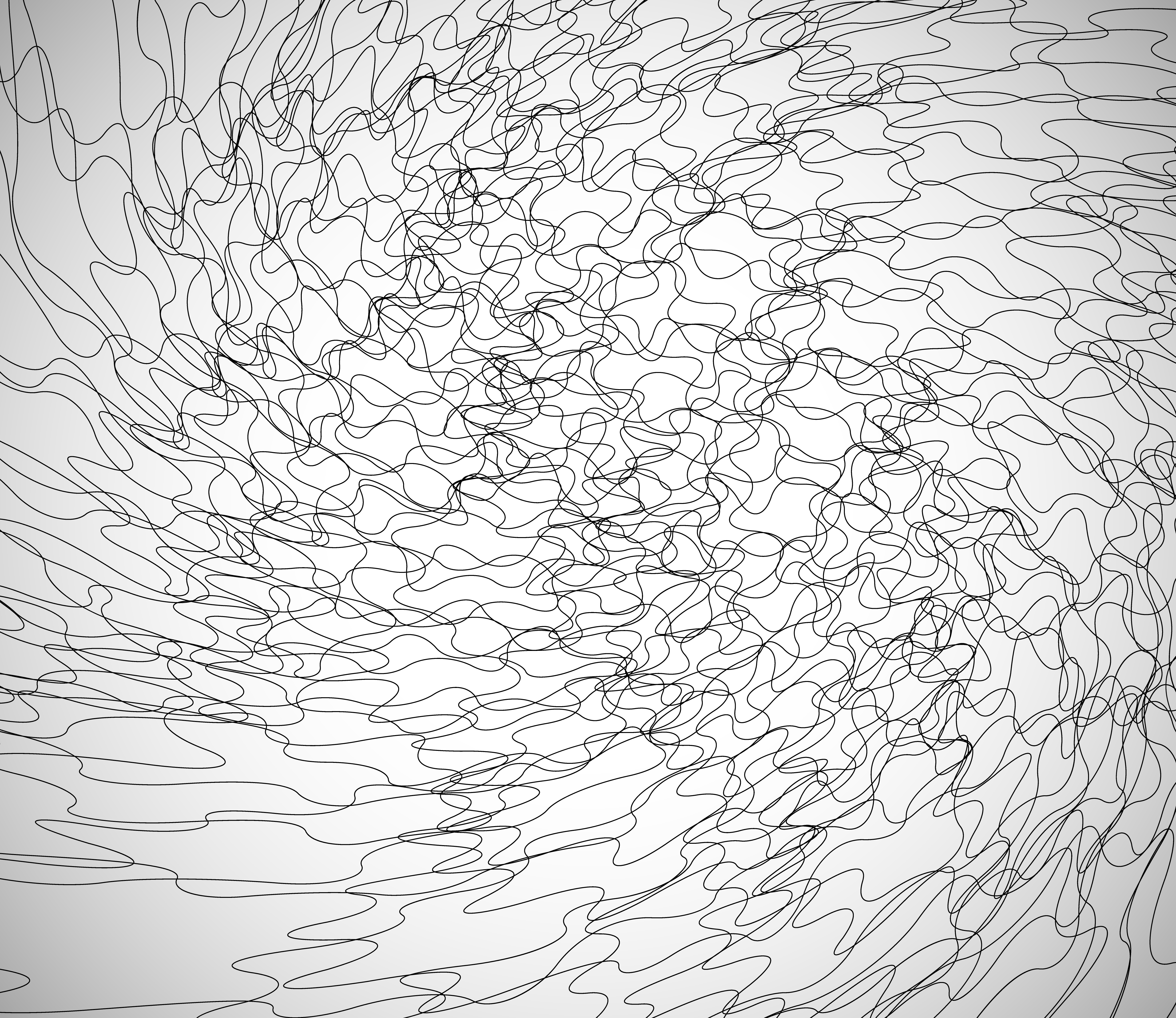
MODS Program in the Foundations and Applications of Mathematical Optimization and Data Science
Institute for Data, Intelligent Systems, and Computation (I-DISC)
Institute for Cyber Physical Infrastructure & Energy (I-CPIE)